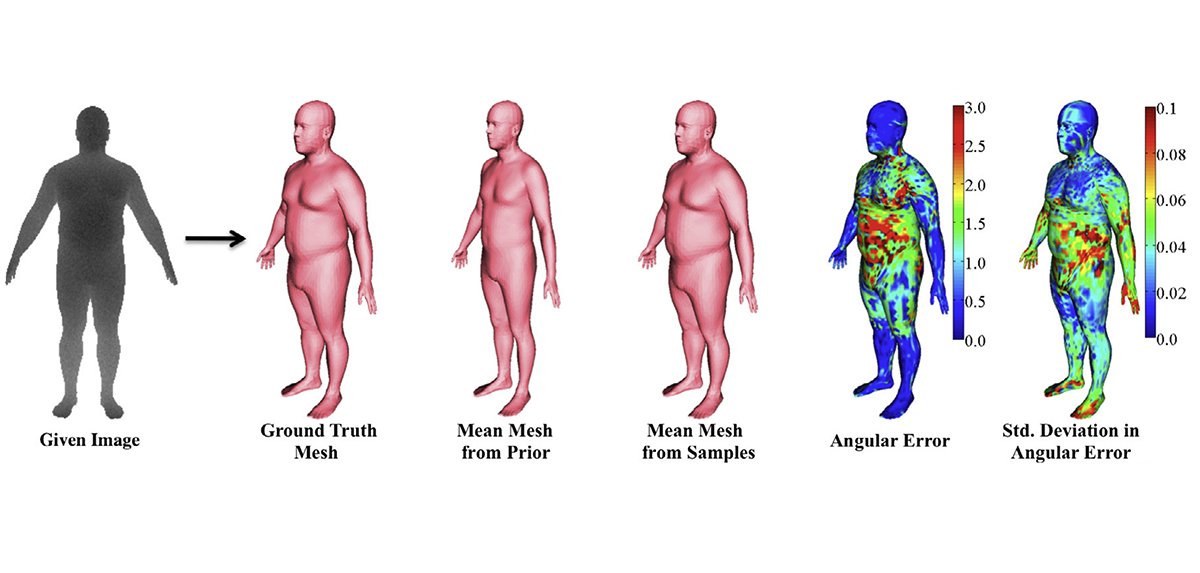
October 16, 2016 The informed sampler: A discriminative approach to Bayesian inference in generative computer vision models
Computer vision is hard because of a large variability in lighting, shape, and texture; in addition the image signal is non-additive due to occlusion. Generative models promised to account for this variability by accurately modeling the image formation process as a function of latent variables with prior beliefs. Bayesian posterior inference could then, in principle, explain the observation. While intuitively appealing, generative models for computer vision have largely failed to deliver on that promise due to the difficulty of posterior inference. As a result the community has favored efficient discriminative approaches. We still believe in the usefulness of generative models in computer vision, but argue that we need to leverage existing discriminative or even heuristic computer vision methods. We implement this idea in a principled way in our informed sampler and in careful experiments demonstrate it on challenging models which contain renderer programs as their components. The informed sampler, using simple discriminative proposals based on existing computer vision technology achieves dramatic improvements in inference. Our approach enables a new richness in generative models that was out of reach with existing inference technology.
Reference
Jampani, V., Nowozin, S., Loper, M., Gehler, P.
In Computer Vision and Image Understanding, Special Issue on Generative Models in Computer Vision and Medical Imaging, 136, pages: 32-44, Elsevier, July 2015